You’ve seen what AI can do. It’s automating workflows, surfacing insights, predicting customer behavior, and even writing code (careful with that). The buzz around it isn’t hype anymore, it’s starting to feel real. Tangible. Like something your company should already be doing. And if you’re leading a mid-sized business, you’re probably thinking: “Okay, so how do we get started with this?”
You start exploring options. You sit through a few demos, talk to vendors, and read the case studies. Everyone’s promising transformation. Everyone’s platform is “seamless.” But somehow, it doesn’t feel simple. You’re not entirely sure what problem you’re solving, how long it’ll take, or if your team is even ready for it. The excitement turns into doubt. And you’re not alone.
The reality is that AI still feels out of reach for most mid-sized companies. Not because the technology isn’t there, but because between the cost, complexity, talent gaps, and integration headaches, most companies are stuck on the sidelines. Watching the AI revolution happen from a safe distance.
In this piece, we’re going to break that down. No hype, no fearmongering. Just a real look at the AI adoption challenges that mid-sized companies are facing today and how a smarter, more flexible approach can make it not only accessible but useful.
Blog Summary:
The potential of AI is there, the tools are everywhere, and the pressure to keep up is real. But when it’s time to move from interest to execution, most teams hit a wall.
What you’ll learn:
- What myths are stopping mid-sized companies before they even get started
- Why having data isn’t the same as being ready for AI
- The hidden costs of generic tools that don’t really fit
- How AI creates a growing advantage for early adopters
- Why it’s not about building an AI department, but building the right solution
- What mid-sized companies can do right now
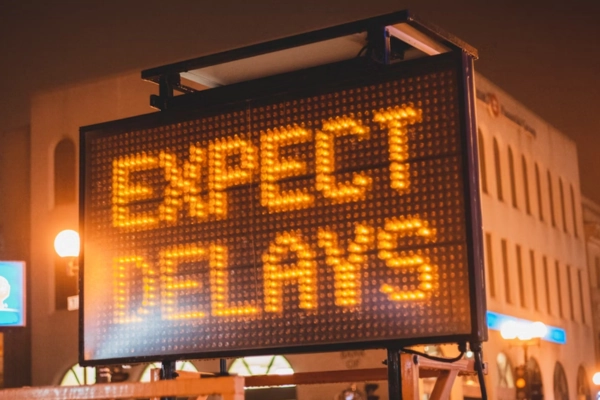
Table of Contents:
- Blog Summary:
- Real Interest, Real Roadblocks
- Misconception #1: AI Means a Giant Investment
- Misconception #2: You Need Massive Data Lakes
- Internal Teams Might Not Be Ready and That’s OK
- One-Size AI Tools Don’t Fit Anyone
- The Innovation Gap Is Growing
- An AI Feature Isn’t an AI Strategy
- Custom Development: The Secret Weapon
Real Interest, Real Roadblocks
Just to be clear: mid-sized companies aren’t ignoring AI. The opposite, actually. They’re asking the right questions. They’re sending links around, sketching ideas on whiteboards, and trying to figure out where AI could make a real impact. But good intentions meet friction fast.
The deeper they dig, the messier it gets. Which models make sense? Is the data even usable? How much will this cost us and how long until we see value? What begins as a bold initiative ends up buried under technical questions no one on the team feels confident answering.
So progress slows. Projects stall. Or worse, they launch something generic just to check the “AI” box. And the real potential? Still sitting on the sidelines. Still “maybe next quarter.” These early efforts often stall due to common AI adoption challenges.
Misconception #1: AI Means a Giant Investment
One of the biggest blockers is also one of the most common myths. To adopt AI, you need a seven-figure budget, a team of machine learning experts, and the kind of infrastructure only enterprise giants can afford. That belief alone is one of the more persistent AI adoption challenges. Enough to stop most mid-sized companies in their tracks.
It’s not hard to see why. The media loves to spotlight massive AI investments. $100M in infrastructure here. A new division with 80 researchers there. It paints a picture of AI as something exclusive, expensive, and way out of reach for anyone not sitting on venture capital or Fortune 500 revenue.
The reality is more nuanced. Yes, building an internal AI team from scratch is costly. And yes, throwing AI at a problem without a plan can lead to wasted time and budget. But smaller, focused AI solutions do exist. You don’t need to boil the ocean to see value. You need to solve the right problem, with the right scope, and the right team.
Misconception #2: You Need Massive Data Lakes
Another common belief: AI only works if you have mountains of data. Clean, structured, centralized. The kind of data setup that most mid-sized companies simply don’t have. This idea pushes a lot of companies out of the conversation before they even begin.
It’s true that AI needs data. But it doesn’t need all the data. It needs the right data. In many cases, smaller, focused datasets are enough to train useful models. Especially when you’re solving a specific problem and not trying to recreate OpenAI.
Mid-sized businesses often underestimate what they already have. Sales logs, support tickets, and product usage patterns. The data is there, even if it’s a bit messy. The challenge isn’t the size. It’s knowing how to extract value from it.
Internal Teams Might Not Be Ready and That’s OK
It’s a fair concern if your team is not used to AI. Most mid-sized companies don’t have AI expertise in-house. No data scientists, no ML engineers, no one who’s built a model before. And that makes AI feel off-limits from the start.
It’s also a normal concern. AI is still new territory for a lot of teams. Expecting your existing developers or product managers to suddenly handle machine learning pipelines isn’t realistic. And hiring for it is tough. Talent is scarce, expensive, and usually drawn to bigger names or R&D-heavy companies.
The lack of internal AI skills doesn’t mean you can’t adopt AI. It just means you need to approach it differently. With the right external team, your company can focus on the problem while someone else handles the complexity.
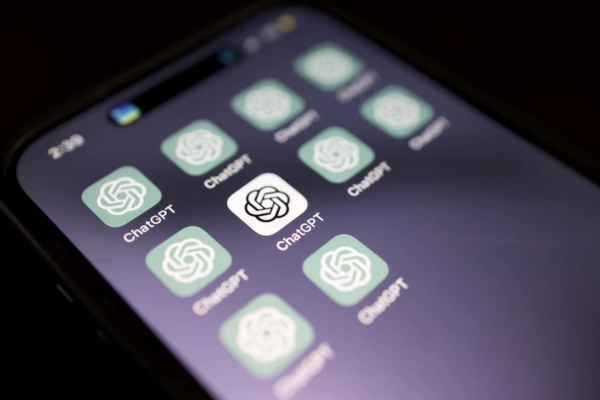
One-Size AI Tools Don’t Fit Anyone
There’s no shortage of off-the-shelf AI tools. Most of them promise quick wins. Pre-trained models, drag-and-drop interfaces, minimal setup. On paper, they sound like a dream. In practice, they rarely deliver.
Let’s say your company finds an AI tool that looks promising. It solves a real problem, has solid results, and the pricing makes sense. There’s just one issue: getting it to work as you want it to with your current systems.
Generic tools don’t know your workflows. They don’t speak your data language. They weren’t built for your customers, your team, or your business model. So, what ends up happening is a forced fit. Teams spend more time adapting to the tool than the tool spends solving the problem.
And when results fall short, the conclusion is usually that AI doesn’t work. When the real issue is that the solution was never built for them in the first place. Off-the-shelf sounds easy. But easy doesn’t scale.
The Innovation Gap Is Growing
Startups are moving fast. Enterprises are pouring money into R&D. Mid-sized companies are stuck in the middle. They’re not small enough to pivot overnight. They’re not big enough to throw budget at experiments. So they wait, evaluate, and reconsider. And while they do, the gap widens.
AI isn’t just a competitive edge anymore. It’s becoming the baseline. Companies that adopt it early are learning faster, operating leaner, and getting ahead. The ones that don’t risk falling behind. This of course is not because they’re doing something wrong, but because others are moving faster.
Just know that the longer AI stays on the roadmap instead of the stack, the harder it becomes to catch up. Because while you’re evaluating, others are iterating. They’re learning what works, what doesn’t, and where the real value is. They’re building internal knowledge, cleaning up their data, and shaping their operations around new capabilities. That momentum compounds over time.
An AI Feature Isn’t an AI Strategy
One of the most common mistakes is treating AI like a checkbox. Add a chatbot. Plug in a recommendation engine. Drop an integration that says “AI-powered” and call it a day. That’s not a strategy. That’s decoration.
AI works when it’s tied to a specific objective. When it’s built around the way your business operates, the data you already have, and the outcomes you’re trying to reach.
Too often, companies jump straight into tools without stepping back to ask the real questions. What are we trying to improve? What decisions could be automated? Where is the friction in our workflows? AI should serve those answers not distract from them. If the value isn’t clear before implementation, it won’t be clear after either.
Custom Development: The Secret Weapon
If building an internal AI team isn’t realistic, and off-the-shelf tools fall short, the next question is obvious: then how? The answer is a model that’s already working for mid-sized companies in other areas: a proven way to overcome AI adoption challenges through external software teams with the right experience, brought in to solve a real problem, not to play innovation theater.
Custom software development backed by SaaS thinking means faster execution, less overhead, and no need to reinvent the wheel. You get a solution that fits your business, your data, and your goals. Not someone else’s template.
It also means flexibility. You don’t need a full-time AI team. You need a team that already knows how to work with your stack, your people, your pace, and who can help you move forward without getting buried in complexity. That’s where we come in.
At CodingIT, we help companies like yours bridge the gap between ambition and execution. We build software teams that understand business goals first, and we deliver AI where it actually makes sense: with results, not buzzwords. Let’s talk about what you’re trying to build. And let’s build it right.