Every day, there’s a breakthrough in Artificial Intelligence. A fresh startup promising to revolutionize entire industries, or a bold claim that AI will replace entire workforces. Investment dollars are flowing in at an unprecedented rate, and AI-powered tools are becoming part of everyday workflows in ways that would have been unthinkable just a few years ago.
At the same time, skepticism is growing. Some experts are questioning whether the industry’s momentum is sustainable, pointing to skyrocketing valuations, overpromising startups, and the sheer volume of funding pouring into AI without clear revenue models. The conversation about an “AI bubble” is gaining traction, with comparisons to past tech booms, some of which ended in rapid corrections.
But is AI heading toward a collapse, or is this just a normal cycle of innovation playing out in a particularly high-stakes industry? The reality, as always, seems more nuanced. While speculation and hype exist, AI’s practical applications are also transforming businesses, increasing efficiency, and reshaping industries. Some AI-powered solutions are already proving indispensable, while others still feel like concepts searching for a market.
The discussion shouldn’t be about whether AI is disappearing but about how companies, investors, and developers navigate its evolution. Market fluctuations are inevitable, but long-term adoption is driven by execution, not speculation.
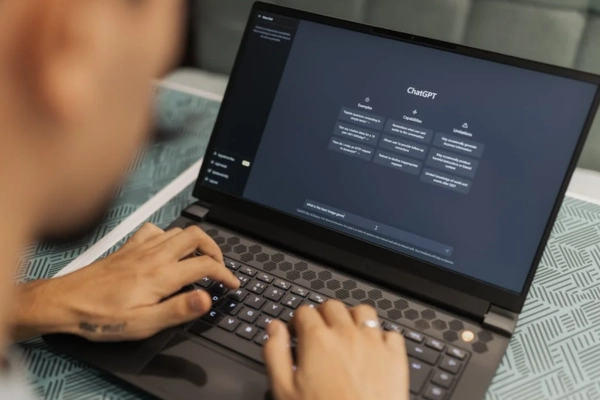
The AI Boom
Some might doubt it, but AI isn’t new. The concepts behind it trace back decades, and major breakthroughs in deep learning, neural networks, and computing power have been in the works for years. What’s changed recently is AI’s commercial explosion: a shift from research labs to boardrooms, from academic theory to billion-dollar product launches.
Unlike some past tech trends, AI’s current boom isn’t happening in isolation. It has been fueled by advances in cloud computing, data availability, and hardware acceleration, which have made AI more practical and accessible. This has resulted in a surge in investment, corporate adoption, and widespread media attention, driving an industry that seems unstoppable.
History shows that rapid technological advancements often involve speculation. The dot-com boom of the late 1990s, for example, saw massive investments in internet companies, some of which changed the world, while others collapsed just as quickly as they appeared. More recently, the crypto and blockchain surge followed a similar trajectory, with projects that were either groundbreaking or unsustainable.
AI’s trajectory may not be identical to these past cycles, but patterns of hype, overvaluation, and market corrections are common in disruptive industries. So, it’s natural to wonder how much of its current growth is built on sustainable, long-term value.
What Is a Tech Bubble?
The term “bubble” gets thrown around whenever an industry experiences rapid growth, but not every surge in investment ends in a crash. A true tech bubble typically follows a familiar pattern: skyrocketing valuations, speculative investments, and unsustainable business models. It’s fueled by excitement, but eventually, reality sets in.
Looking back, the dot-com era is the classic example. Billions poured into internet startups with little more than a domain name and an idea, leading to a wave of bankruptcies when the hype couldn’t sustain itself.
AI has certainly attracted speculation, but does that mean it’s a bubble? Not necessarily. AI is already embedded in real-world applications: powering automation, streamlining operations, and generating measurable value. Of course, some companies may be overpromising what AI can realistically achieve, and the market could correct it in certain areas. But a correction isn’t the same as a collapse. If anything, history suggests that hype alone doesn’t determine whether a technology survives.
The Role of Investors
Massive investments have propelled AI’s rise, but with so much capital flowing in, it’s fair to ask: are investors driving sustainable innovation, or are they inflating expectations?
Venture capital firms and tech giants have poured billions into AI startups, some with groundbreaking ideas and others with little more than a pitch deck and a promise. A mix of genuine breakthroughs, overhyped solutions, and companies chasing funding more than viability have come out of it so far.
History suggests that investor enthusiasm can be a double-edged sword. On one hand, it accelerates development, attracting top talent and fast-tracking new technologies. On the other, it can lead to inflated valuations and unrealistic growth expectations that some companies simply can’t meet.
AI Adoption in Business
Some businesses are leveraging AI to improve efficiency, automate repetitive tasks, and enhance decision-making. Others are integrating AI simply because it’s expected, without a clear understanding of how it fits into their long-term strategy.
There’s a difference between using AI as a competitive advantage and using it as a marketing buzzword. Companies that invest in well-defined, practical applications tend to see real benefits. Meanwhile, those who jump on the AI bandwagon without a plan risk wasting resources on projects that never deliver meaningful results.
It seems natural that businesses that approach it with a clear purpose and realistic expectations will likely see the most long-term success. Those who don’t may end up with expensive tools that look impressive on paper but fail to create actual value.
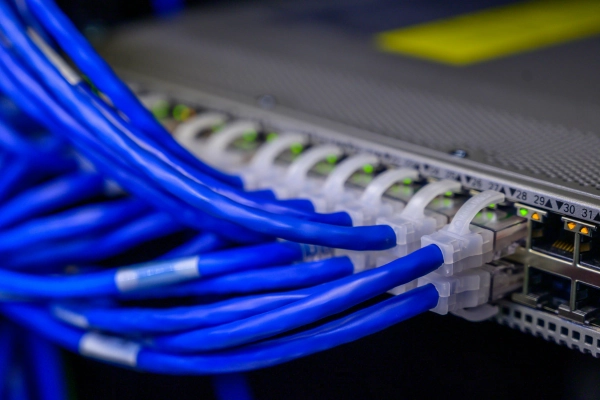
The Challenges AI Faces
While the technology itself is evolving, several practical challenges continue to shape AI’s trajectory. For one, data quality and availability remain critical issues. AI models are only as good as the data they’re trained on, and biased, incomplete, or low-quality datasets can lead to unreliable results.
Computing power is another constraint. Training large-scale AI models require massive infrastructure investments, making it difficult for smaller players to compete. Then there’s the talent gap. The demand for AI expertise far outweighs the supply, making it harder for businesses to find skilled engineers and researchers. As a result, many companies struggle to build AI solutions in-house, relying instead on third-party tools that may not fully align with their needs.
One way businesses overcome these obstacles is by partnering with experienced development teams that can provide the right expertise and tailored solutions. Rather than navigating these challenges alone, companies working with specialized teams can focus on integrating AI in a way that aligns with their business goals.
These limitations don’t make AI any less valuable, but they do highlight the practical realities businesses must navigate. Those who recognize these challenges and plan accordingly will be in a stronger position to integrate AI effectively.
Regulation and Ethics
As adoption skyrockets, so does the attention from governments, regulators, and advocacy groups. Conversations about AI ethics, transparency, and accountability are no longer theoretical.
Regulation isn’t inherently a roadblock to progress, but it does add complexity. Some governments are pushing for stricter oversight, while others are taking a more hands-off approach to encourage innovation. The challenge is striking a balance, ensuring AI remains a powerful tool for progress without creating unintended risks or excessive red tape. How this plays out will influence AI’s trajectory just as much as the technology itself.
Avoiding the AI Hype Trap
Not every AI initiative succeeds, and the difference between success and failure often comes down to execution. While AI is transforming industries, many businesses fall into the trap of rushing into adoption without a clear purpose.
The real challenge is whether companies know how to use AI effectively. Businesses that expect immediate, game-changing results without proper planning often end up with tools that look impressive but fail to deliver.
A smarter approach is to prioritize AI projects with tangible, measurable outcomes. This means identifying areas where AI can create real efficiency gains, integrating it into existing workflows, and ensuring it’s scalable for long-term use.
The Role of Software Development in AI’s Longevity
AI might be getting all the attention, but it’s only as good as the software supporting it. No matter how advanced an AI model is, without a solid foundation of scalable software, reliable infrastructure, and seamless integration, it remains a theoretical solution rather than a functional tool.
Some companies pour resources into AI development while overlooking the engineering required to bring it to life. Data pipelines break, models fail to scale, and usability issues turn promising innovations into abandoned projects.
To make AI truly valuable, businesses need more than just cutting-edge algorithms. They need experienced development teams that can integrate, optimize, and maintain AI solutions over time. Those that get this balance right turn AI into a business asset, rather than just an experiment.
Why the Right Development Partner Is Non-Negotiable
AI projects don’t usually get second chances. A poorly implemented solution can drain resources, disrupt operations, and even damage a company’s reputation. The difference between an AI success story and a costly failure often comes down to one critical factor: who is building it. When AI is handled by teams without deep technical knowledge and real-world experience, the result is often an expensive experiment rather than a functional solution.
At CodingIT, we don’t just develop, we make it work for your business. From custom software development solutions to seamless integration, we ensure real, measurable results. Don’t leave your AI investment to chance. Get in touch with us today.